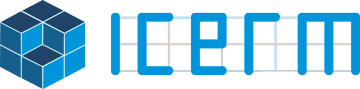
Exploiting Combinatorial Regularity for Topological and Morse Measures of ReLU Neural Networks
Identifying the capabilities of a machine learning model before applying it is a key goal in neural architecture search. Too small of a model, and the network will never successfully perform its task. Too large of a model, and computational energy is wasted and the cost of model training may become unbearably high. While universal approximation theorems and even dynamics are available for various limiting forms of ReLU neural networks, there are still many questions about the limitations of what small neural networks of this form can accomplish. Marissa Masden seeks to understand ReLU neural networks through a combinatorial, discrete structure that enables capturing topological invariants of structures like the decision boundary.
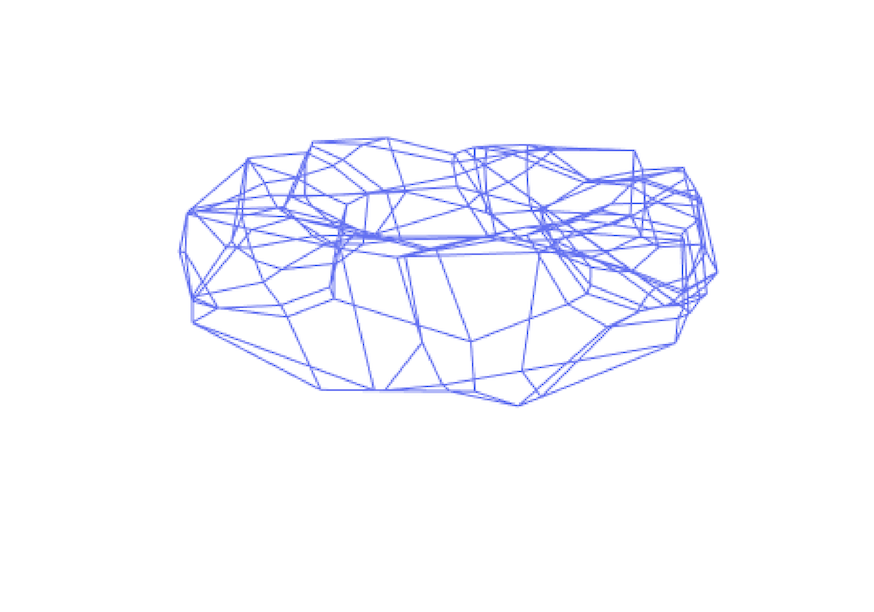
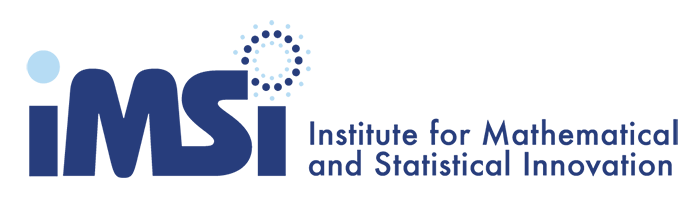
Our Changing World: Algebraic Statistics for Evolutionary Biology and Ecology
Understanding the evolutionary history of a collection of species, through fields such as phylogenomics and comparative phylogenetics, is crucial as we consider the future effects of climate change. Algebraic statistics provides algebraic and geometric tools to study the models commonly used in evolutionary biology. The Institute for Mathematical and Statistical Innovation (IMSI), hosted a workshop, “Algebraic Statistics for Ecological and Biological Systems,” as part of a Long Program on “Algebraic Statistics and Our Changing World,” which highlighted these connections.
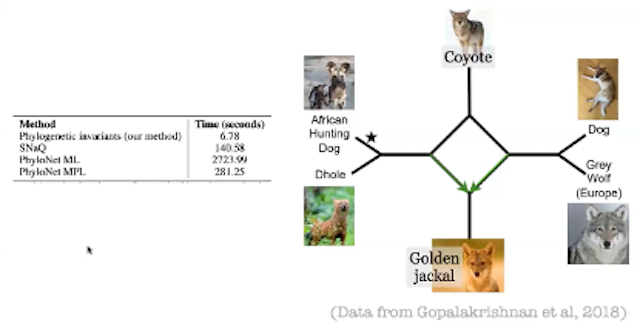
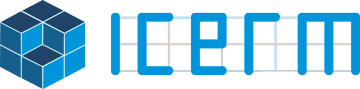
Improving Newton’s Method Near Singular Points with Anderson Acceleration
Nonlinear equations are ubiquitous in the sciences, a famous example being the Navier-Stokes equations in fluid mechanics. To compute a numerical solution for a given nonlinear equation, one often employs an iterative scheme such as Newton’s method. Recent works by Matt Dallas, Sara Pollock, and Leo Rebholz analyze Anderson accelerated Newton’s method applied to singular problems.
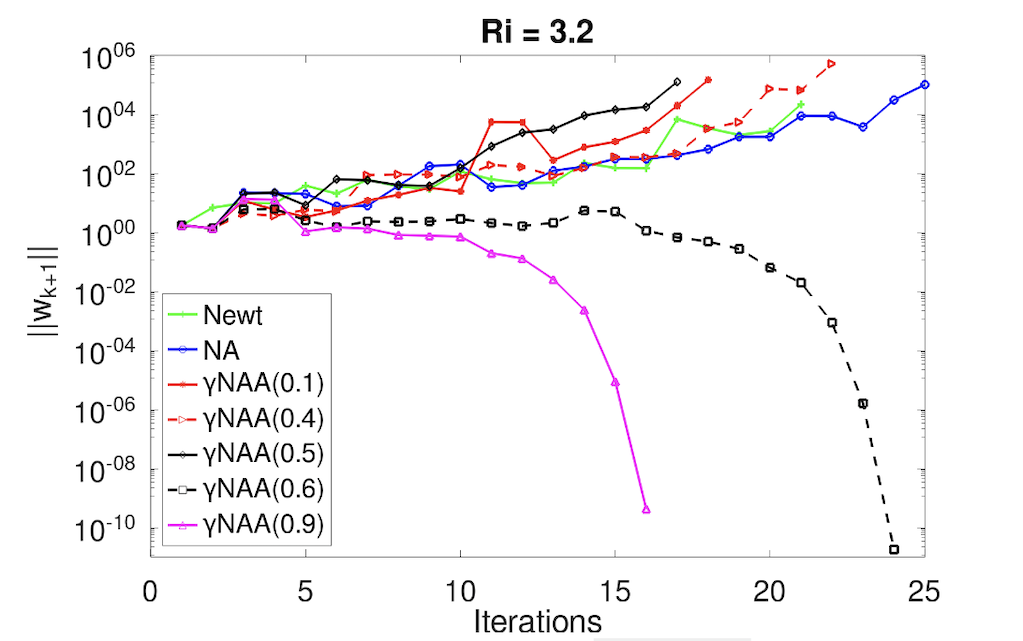
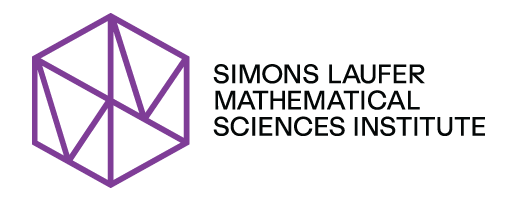
Euler Systems
In the mid-19th century, while attempting to prove Fermat’s last theorem, German mathematician Ernst Kummer started investigating arithmetic on novel number systems.
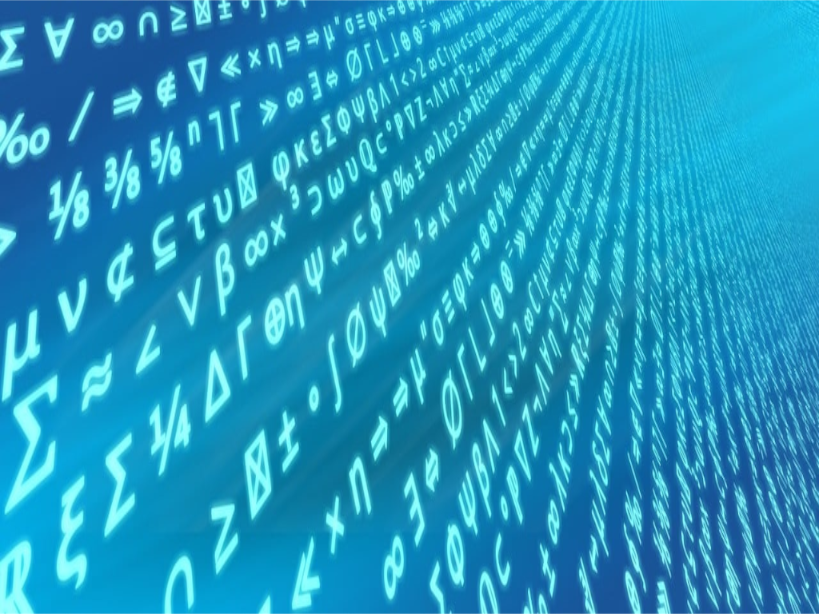
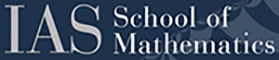
Traffic and Theory: Waves on Roads and Blackboards
The largest live autonomous vehicle traffic experiment ever conducted began the week of November 18, 2022, in Nashville, Tennessee. It involved 100 cars and a workforce of more than 250, around 70 of whom were researchers. One of the goals of the experiment was to analyze how level two autonomous vehicles (think cruise control with a couple added functions, like speed adjustment that uses LIDAR) can impact traffic waves, specifically those representing frustrating “stop and go” conditions...
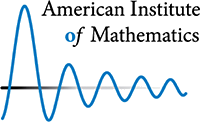
Higher uniformity of bounded multiplicative functions
Kaisa Matomäki, Maksym Radziwill, Terence Tao, Joni Teräväinen, and Tamar Ziegler make progress on the conjectures of Sarnak and Chowla with their paper “Higher uniformity of bounded multiplicative functions in short intervals on average” published in the Annals of Mathematics in 2023. The work originated in a working group at the AIM workshop “Sarnak's conjecture” in December 2018.
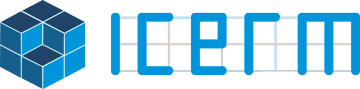
Giving a Quadratic Solution to the Word Problem in 3-free Artin Groups
Artin groups emerged in the 1960s as generalizations of braid groups and modifications of Coxeter reflection groups involving the removal of the involution relation inherent in reflections, thus creating groups that project onto Coxeter groups. For example, braid groups with n strands project onto the symmetric group of n elements. Therefore, Artin groups have a very simple presentation: they are groups generated by a finite set of generators S and relations written as sts · · · = tst . . . for s, t ∈ S, where both words in the equality have the same length. However, groups so simple to define do not admit many common techniques that allow obtaining global results for any Artin group, making them highly enigmatic groups.
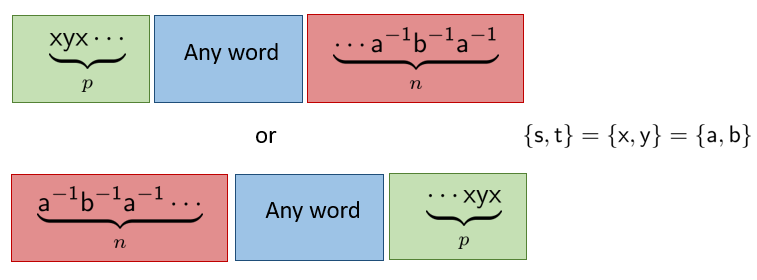
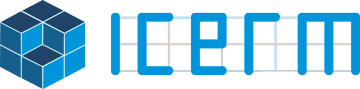
Decoding Neural Representation of Navigation by Mathematical Modeling and Quantitative Data
The navigational behavior of an animal consists of several steps of information processing, including the detection of the surrounding environment, integration of multi-modal sensation, and generation of actions. Whole steps are computed in a neural circuit, or a group of interconnected neurons that work together to carry out a specific function. Understanding how each navigation process is implemented in a neural circuit is a big challenge in neuroscience. Because ensembles of the neural dynamics are only partially observed due to technical limitations, a combination of theoretical and experimental approaches is necessary to tackle this challenge.
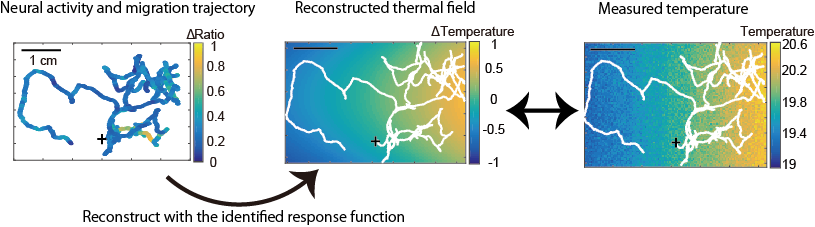
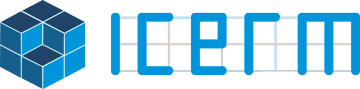
Interpreting the Shape of Neural Activity
An animal’s brain encodes representations of important aspects of the world and its own internal state through its constituent neurons. This information is distributed across many different anatomical regions in the brain, each of which performs computations and passes the results to others, with the end result producing behavior. The foundational observation in topological data analysis, or the study of datasets’ geometric features, is that the coarse, mesoscale structure of data can be described in terms of circles, spheres, tori, and a galaxy of more esoteric objects.
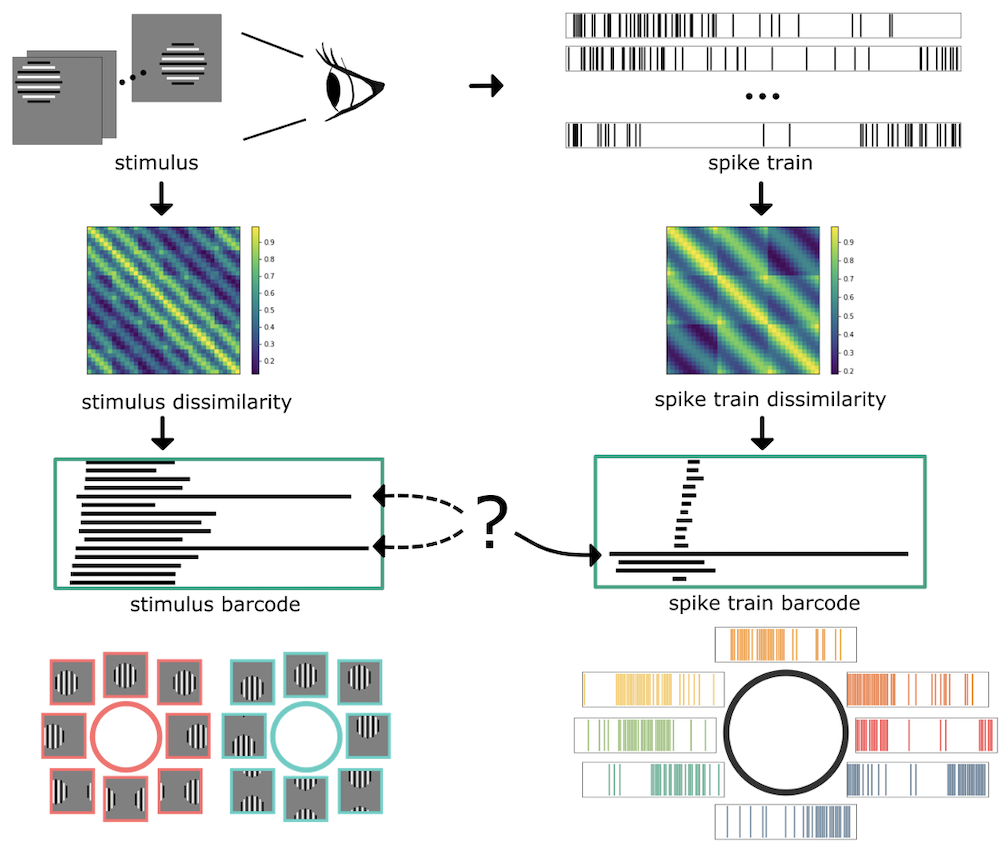
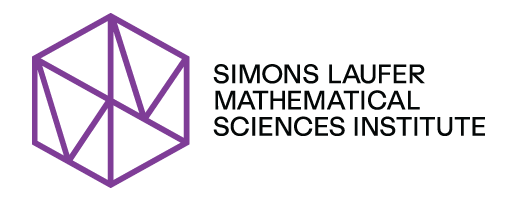
Connections in Gauge Theory
Inspired by the rise of mirror symmetry in theoretical physics, Jason Lotay is investigating connections between mathematical objects called calibrated submanifolds and instantons.
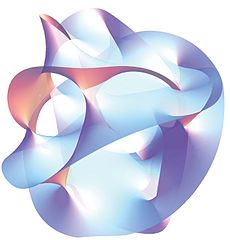