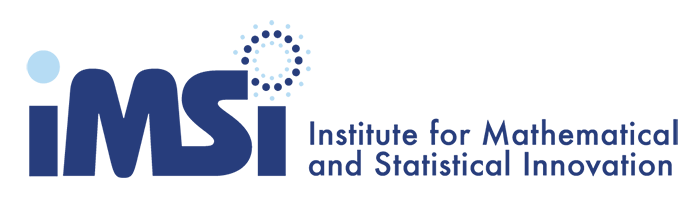
Machine Learning and Carbon Capture
IMSI - January 2025
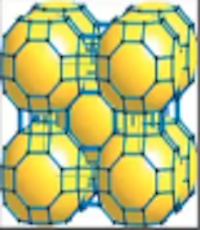
Chemical engineers are teaming up with data scientists to use machine learning as a transformative technology in the discovery of new materials that can be used to capture the carbon dioxide produced by power plants. Their target are metal-organic frameworks – crystalline materials that act like sponges that can selectively absorb greenhouse gases in the flues of coal-burning and natural gas-burning power plants. For example, it was recently announced that ExxonMobil will soon be using natural gas power plants to generate electricity that they would sell directly to data centers. Data centers are extraordinary consumers of electricity, ironically partly due to the vast amount of energy needed for the computations driving machine learning and artificial intelligence. ExxonMobil claims that they will capture 90% of the CO2 produced for powering data centers.
Metal-organic frameworks (MOFs) are three-dimensional porous crystalline materials composed of metal clusters linked by an organic molecule (See Figure 1). Different combinations of metal clusters and the organic linkage that forms the framework structure have resulted in the order of 100,000 materials in the chemical databases. This vast array of MOFs means that chemical engineers are challenged to design MOFs with desired physical properties such as pore size. MOFs are popular in gas separation applications, most notably, selectively capturing carbon dioxide (CO2) from the flue gases in coal- and natural gas-burning power plants. Because of the potential climate impact of MOF technology, chemical engineers are partnering with data scientists who are experts in artificial intelligence and machine learning (ML) to devise techniques for designing MOFs that are engineered to capture CO2, given the highly complex combination of flue gas temperature, composition, flow rate, and volume. Addressing this array of scientific, mathematical, statistical challenges was just one topic addressed in the Spring 2024 Long Program on “Data-Driven Materials Informatics,” hosted by the Institute for Mathematical Statistical Innovation, March 4 - May 24, 2024 at the University of Chicago.
Berend Smit, of the Ecole Polytechnique Fédérale de Lausanne (EPFL), spoke about, “Big Data in Nanoporous Materials Design: Science Beyond Understanding,” during the Long Program’s embedded workshop on Machine Learning Electronic-Structure Theory, March 25-29, 2024. Smit described the broad societal goal of “net zero,” whereby the global outputs of CO2 are balanced (or better) by an array of technologies for carbon capture or sequestration. A major global source of CO2 is energy production, with coal and natural gas being major fuel sources for power plants in most industrialized nations, and the production of steel and cement. As nations make the transition away from carbon-based fuels to green energy, there is increasing demand for energy efficient, cost effective, and clean technologies for capturing CO2 at its source in the energy production process.
Metal organic frameworks are one of the hot technologies currently in use for capturing CO2 from flue gasses, and chemical engineers are challenged to come up with increasingly effective MOFs, based on a set of key criteria, including: 1) material characterization in terms of how well that material selects for capturing CO2 vs other flue gases such as nitrogen, 2) process evaluation, 3) techno-economic analysis which quantifies the cost of net carbon avoidance, and 4) life cycle assessment for determining the availability and use of materials resources (metals, minerals) (See Figure 2). One of the main scientific challenges in engineering MOFs is predicting the oxidation state of the MOF and identifying an optimal material for a particular application. Typically, scientists and engineers have deployed density functional theory (DFT) to understand the electronic structure of the material under study, since electronic structure determines the oxidation state. However, density functional theory becomes less accurate as the temperature of the material under study increases. As an alternative, Smit and his colleagues are turning to machine learning (ML) tools to predict the behavior of novel MOFs, based on training data derived from the open literature.
Chemical databases catalogue the crystal structures of about 100,000 MOFs, but only about half report the oxidation state in the full chemical name. For training ML models, Smit and colleagues use the metal’s location on periodic table, oxidation state data, local chemistry, and coordination geometry. There are about three commonly used ML models and Smit’s team has each model predict an oxidation state for a new MOF and then they compare the outputs of each model. The oxidation state most commonly predicted by the different ML models is the one used for the new material under study.[1]
Machine learning models can also be used for predicting the heat capacity of MOFs, which is important because heat capacity is an important factor in the energy requirement of a candidate MOF for carbon capture. Regeneration is when the captured CO2 is released from the MOF and sequestered elsewhere, so that the MOF can continue to be used for carbon capture in the flue. In the past, heat capacity has been estimated using exact DFT calculations. Yet, solving those equations computationally for a typical 200 atom MOF goes as N^4, making such solutions computationally expensive and time consuming. Smit and his colleagues are now using ML-based heat capacity predictions[2] to better estimate the energy costs of a carbon capture process.[3]
Finally, looking ahead to a future that is fast upon us, Smit and his team are exploring the use of ChatGPT and other large language models (LLMs) for predictive chemistry and materials science.[4] The enabling factor here is that most chemical names and experimental descriptions of these materials are catalogued in text form. Smit cautions, however, that while LLMs are trained on publicly available searches of text, much of the reliable and up-to-date data is in the scientific literature and kept behind publishers’ paywalls. Therefore, if chemists are going to use LLMs in their research, they need to think critically about how the LLMs are trained and on what data in order to understand how LLMs can succeed and fail. And along these lines, Smit urges chemists to make their data freely available so that ML- and LLM-driven chemistry and materials science can have a better chance of yielding useful science.
The convergence of chemistry with data science is rapidly producing new computational tools that chemical engineers will use to create new materials that can assist in carbon capture and sequestration, thus making today’s energy cleaner as economies transition to greener technologies.
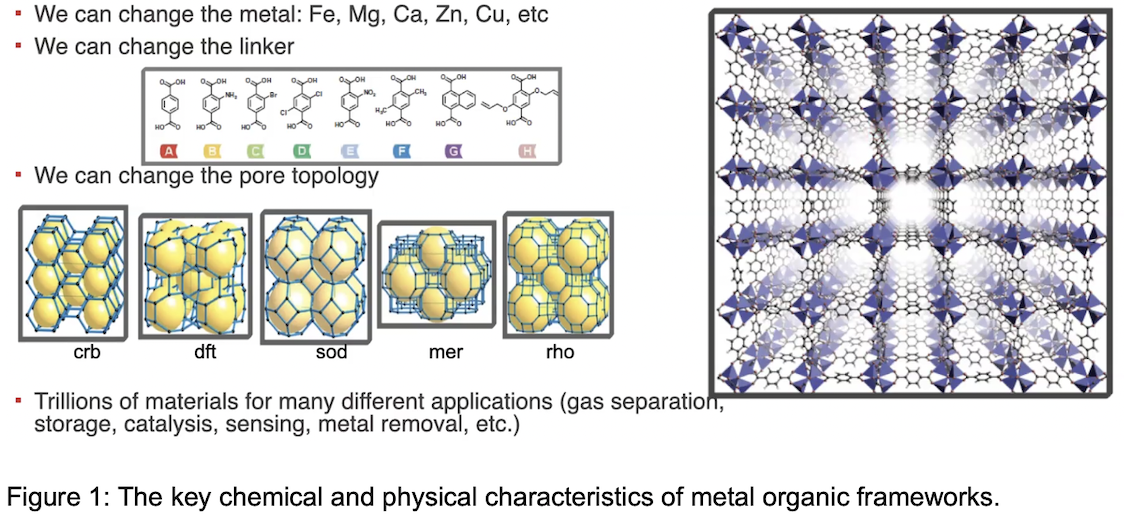
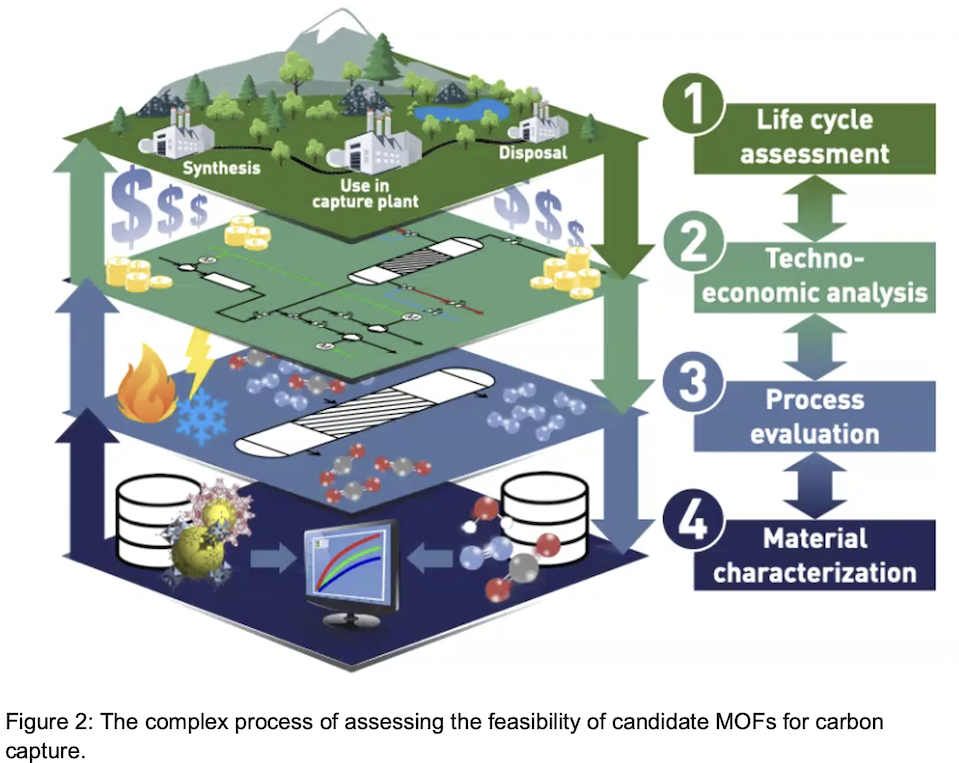
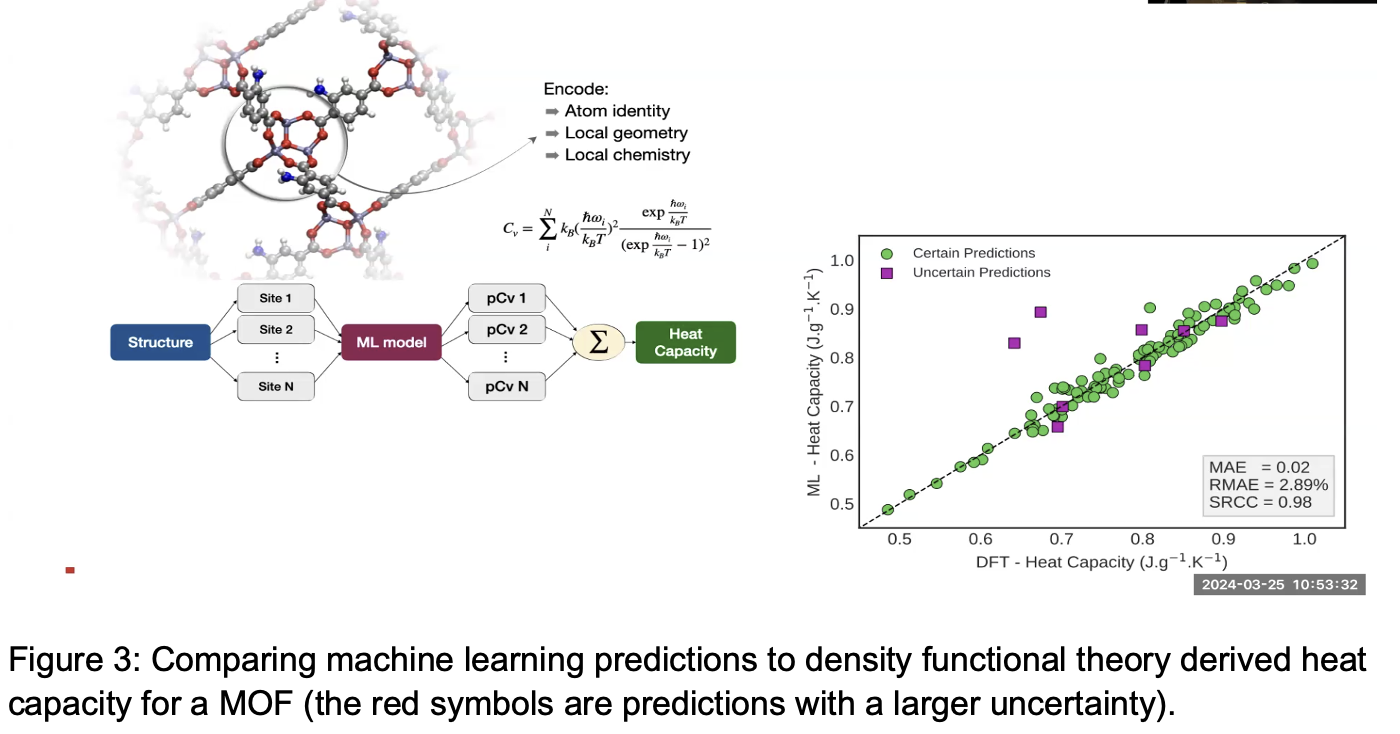
[1] K. M. Jablonka, D. Ongari, S. M. Moosavi, and B. Smit, Using collective knowledge to assign oxidation states of metal cations in metal-organic frameworks Nat. Chem. 13, 771 (2021) http://dx.doi.org/10.1038/s41557-021-00717-y
[2] S. M. Moosavi, B. Á. Novotny, D. Ongari, E. Moubarak, M. Asgari, Ö. Kadioglu, C. Charalambous, A. Ortega-Guerrero, A. H. Farmahini, L. Sarkisov, S. Garcia, F. Noé, and B. Smit, A data-science approach to predict the heat capacity of nanoporous materials Nat Mater 21, 1419 (2022) http://dx.doi.org/10.1038/s41563-022-01374-3
[3] C. Charalambous, E. Moubarak, J. Schilling, E. Sanchez Fernandez, J.-Y. Wang, L. Herraiz, F. Mcilwaine, Shing Bo Peh, Matthew Garvin, K. M. Jablonka, S. M. Moosavi, J. Van Herck, Aysu Yurdusen Ozturk, Alireza Pourghaderi, A.-Y. Song, G. Mouchaham, C. Serre, Jeffrey A. Reimer, A. Bardow, B. Smit, and S. Garcia, A holistic platform for accelerating sorbent- based carbon capture Nature 632, 89 (2024) http://dx.doi.org/10.1038/s41586-024-07683-8
[4] K. M. Jablonka, P. Schwaller, A. Ortega-Guerrero, and B. Smit, Leveraging large language models for predictive chemistry Nat Mach Intel 6, 161 (2024) http://dx.doi.org/10.1038/s42256-023-00788-1