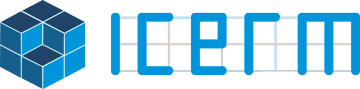
Interpreting the Shape of Neural Activity
ICERM - December 2023
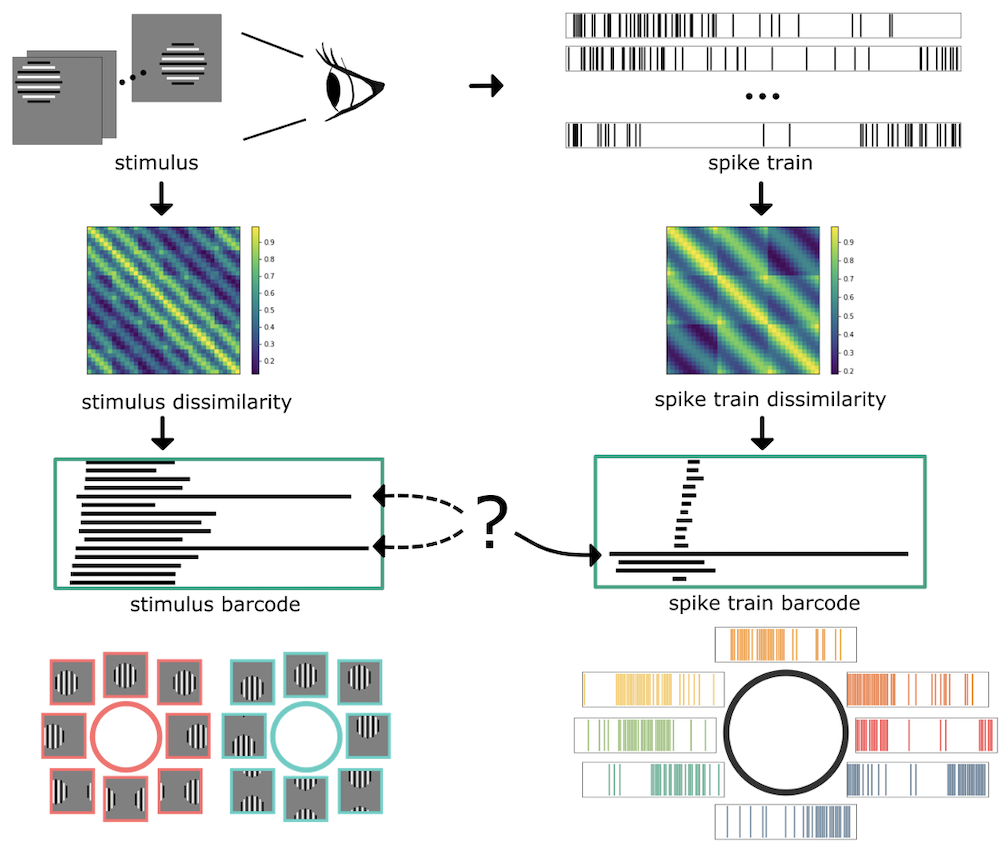
by Chad Guisti and Iris Yoon
An animal’s brain encodes representations of important aspects of the world and its own internal state through its constituent neurons. This information is distributed across many different anatomical regions in the brain, each of which performs computations and passes the results to others, with the end result producing behavior. The foundational observation in topological data analysis, or the study of datasets’ geometric features, is that the coarse, mesoscale structure of data can be described in terms of circles, spheres, tori, and a galaxy of more esoteric objects. Such shape descriptors have been in heavy use by neuroscientists for decades, dating back at least to Hubel and Wiesel’s Nobel Prize-winning discovery of receptive fields in the visual cortex of cats.
However, simply detecting a shape that describes how the observed activity of a population of neurons is organized is not sufficient for understanding the function the population is performing. We must also assign semantics to the shape in terms of something we already understand. In the case of neuroscience, this could involve some aspect of the environment or behavior, or neural activity in another, better-understood anatomical region.
Classical algebraic topology provides tools for assigning such semantics in the form of induced maps on homology: given two shapes, a continuous function from one to the other descends to the level of such algebraic invariants, for example providing a linear map between the associated vector spaces. If we know how to interpret one of the shapes, we can use the data of an induced map to study the other.
However, in the data context, we do not have access to the shapes, much less such a continuous function. Nor, in many cases involving spaces constructed to model neural population activity, should we expect such a function to exist. We often observe a weighted relation between activity among units in different populations; any measure of time series similarity will suffice.
In our recent paper “Persistent Extensions and Analogous Bars: Data Induced Relations Between Persistence Barcodes,” by Iris H.R. Yoon (Wesleyan University), Robert Ghrist (University of Pennsylvania), and Chad Giusti (Oregon State University), we develop the mathematical and computational methods needed to construct relations on the persistent homology of related shapes using the data of a cross-similarity measure. In forthcoming work, Yoon and Giusti, along with collaborators Spencer Smith and Yiyi Yu, apply these methods to track cyclic structure across simulated and in vivo neural populations in the primary visual cortex.
This work is being developed at ICERM during the Fall 2023 Semester Program Math + Neuroscience: Strengthening the Interplay Between Theory and Mathematics.