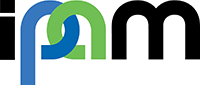
AI for Chemical Design
Exploration of chemical compound space unites quantum chemistry with artificial intelligence
By combining quantum chemistry with artificial intelligence (AI) or Machine Learning, core participants of the long program, “Navigating Chemical Compound Space for Bio and Materials Design”, achieved a scientific breakthrough expected to aid in exploring chemical compound space, i.e. the virtual space populated by all possible chemical compounds.
The interdisciplinary team from the Technische Universität Berlin (Germany), the Fritz-Haber Institute of the Max-Planck Society (Germany), and the Argonne Leadership Computing Facility (United States) forged a collaboration at IPAM that promises to dramatically increase the speed of calculating energies of small molecules with quantum chemical accuracy. Quantum chemical methods permit scientists to calculate molecular properties on a computer from first principles (i.e. without having to conduct any experiments) — they provide essential insights and understanding of many chemical processes including catalysis, drugs, or battery materials. Previously, such calculations demanded intensive computational resources.
By contrast, Machine Learning generates predictive models based on examples. While common in daily life, such as in Google’s internet search engines or Amazon’s book suggestions, it is also used in scientific domains, such as genetic research or brain computer interfaces. When applied to quantum chemistry, thousands of quantum chemical reference energies have been calculated in order to “learn” a molecular model. The resulting Machine permits the prediction of molecular properties with comparable accuracy within milliseconds, instead of hours. Such speed-up paves the way for highly accurate calculations of unprecedentedly many molecules.
Originally published in: Matthias Rupp, Alexandre Tkatschenko, Klaus-Robert Müller, and O. Anatole von Lilienfeld, Fast and Accurate Modeling of Molecular Atomization Energies with Machine Learning, Physical Review Letters 108, 058301 (2012); doi:10.1103/PhysRevLett.108.058301.
Normalized distribution of distances between molecules
in the chemical space that was considered for this study